Futurist > Edge of the future > PoE-GAN: NVIDIA’s New Image Synthesis Framework
PoE-GAN: NVIDIA’s New Image Synthesis Framework
Researchers at NVIDIA have revealed details of a new AI image-generation system that makes notable advances on the company’s former tools, such as GauGAN, by combining several methods of user input – text, sketch, and semantic segmentation.
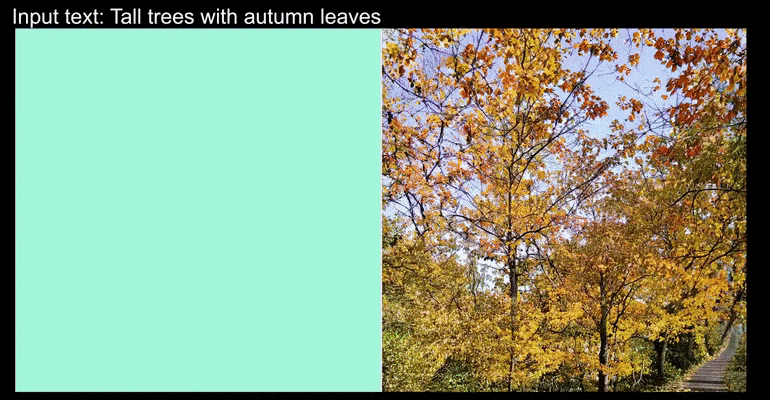
Poe-GAN combines three methods of input for image generation, allowing refinement and direct manipulation. Source: https://arxiv.org/pdf/2112.05130.pdf
Titled PoE-GAN, the new framework – which the authors note uses similar methodologies to a Chinese paper published in May – is capable of generating images from any one of the three modalities, and allowing the user to refine the initial output with additional modalities.
(An associated video demonstrating PoE-GAN has been released – scroll down to check it out, embedded at the end of this article)
For instance, it’s possible to create an image by inputting the text ‘beach with sand and waves’, which will result in a randomly-created image derived from thousands of similar images on which the Generative Adversarial Network (GAN) behind PoE-GAN has been trained.
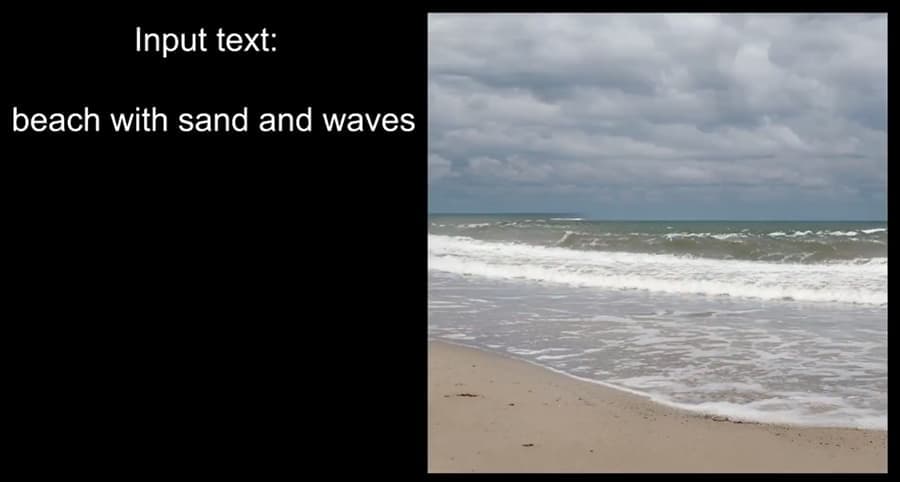
In the ‘text input’ modality, the user describes a scene, and PoE-GAN randomly generates an apposite image.
After this stage, one can refine the image by adding further text inputs, such as ‘with cloudy skies’ or ‘in a storm’. However, this will quite radically change the generated image, rather than adding clouds to the existing one:
But it’s not necessary to begin in this way, or to use text input as the starting point. One can begin by choosing a domain (i.e. ‘landscapes’) and letting PoE-GAN interpret the doodles into a photorealistic image, much as NIVIDIA’s GauGAN has been doing for the last few years.
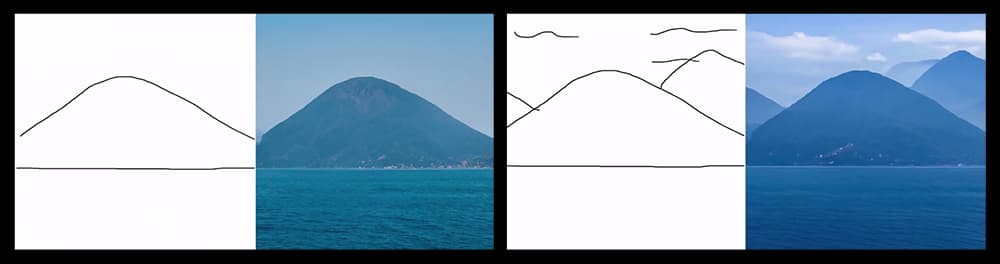
PoE-GAN, like its predecessor GauGAN, can dynamically interpret crude sketches into detailed and photorealistic scenes.
Finally, instead of drawing crude lines, we can also draw in large areas of a certain type of content using semantic segmentation – large swatches of color that ‘stand in’ for a domain, such as sea, shore, tree, mountain, etc.
In the video’s example of multimodal input, which uses all three types of input to create and refine an image, we see the user address a specific domain with the input Tall trees with autumn leaves.
A base, random image appears, indicating that PoE-GAN understands the domain, and that the domain is now ‘set’:
Now we can draw in large patches of color – presets that PoE-GAN has already associated with specific domains, and generate the basis of a persistent image that’s consistent with the domain we selected with the text at the start.
If we hit ‘generate’, with these settings, we’ll get a number of varying outputs that stick to both types of user input – the text Tall trees with autumn leaves and the crudely painted-in areas delineating river, tree, mountain, and sky.
Now we can add a third input, and begin to sketch in specific items, such as ‘mountains’.
Here, we’re altering the outline enough to cause a shift also in parts of the image that aren’t directly affected by the sketch. In the image above, we see that the user has added two daubs for ‘tree’, that are impinging on the already-defined tree region, which cause that grouping to shift a little. Additionally, sketching in clouds has changed the scene lighting.
With these more detailed instructions now set in stone, we can once again hit ‘generate’, in order to cycle through various possible synthesized interpretations, which are now far more constrained:
Image Persistence
It’s not clear how possible it will be in later versions to ‘freeze’ sections of an image so that they remain unaffected by subsequent iterations, or by additional user input, since such input has a synergistic effect on the dynamics of the generated image.
Neither does it seem possible, when an image creation process is as far advanced as demonstrated above, to change the root domain, such as changing the original text input from Tall trees with autumn leaves to Tall trees in springtime.
However, the history of GauGAN suggests that PoE-GAN will be made available as a desktop application and in an online version, so presumably these matters will quickly become clear.
These modalities – text, semantic segmentation, and sketch – can be used singly or in any combination in Poe-GAN, and the researchers behind the project report that in single modality mode, PoE-GAN outperforms its limited range of rivals, and achieves better Frechet Inception Distance (FID) scores in this respect.
Details of the new framework are available in the paper Multimodal Conditional Image Synthesis with Product-of-Experts GANs, authored by four researchers at NVIDIA.
Editing Real Images
PoE-GAN can also integrate a real, user-provided photo into its workflow, enabling AI-editing of photos with any of the available tools.
In this case, the framework relies on recognizing domains in the uploaded image and applying automated semantic segmentation to it, instead of the user drawing in the segmentation themselves.
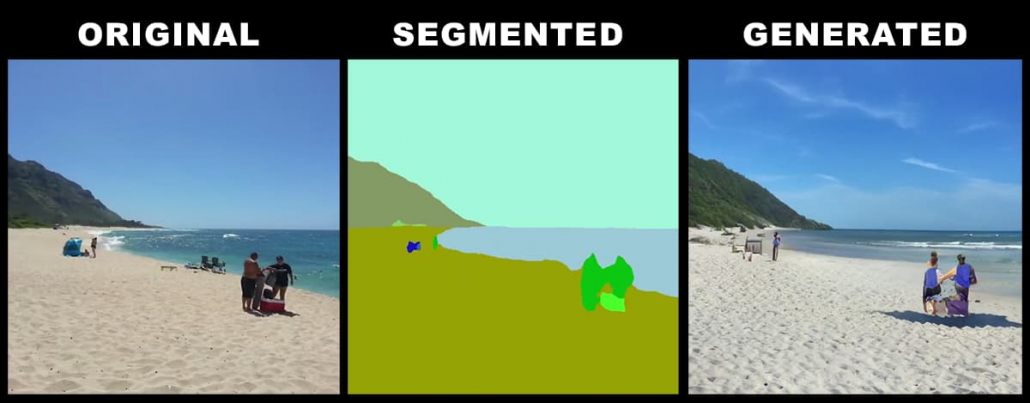
On the left, the original user photo; middle, PoE-GAN’s semantic segmentation, based on recognition of domains; right, a generated image. Note that the people are sketchy, but recognizably people, and that the automated segmentation of them from the original has some ambiguity in the lower region (bright green). The automated semantic segmentation can be edited (see animated image below).
From this point, one can treat of the material quite freely, amending the extracted semantic segmentation mask and applying domains base on a number of input modalities:
This is not the kind of pipeline suitable for removing an ex from holiday pictures, since it takes the original image as a point of departure, leaving it semantically intact but still notably altered throughout.
PoE-GAN is not limited to landscapes. Though its results on other domains are an improvement on the state-of-the-art in multimodal input image synthesis, there’s a way to go yet:
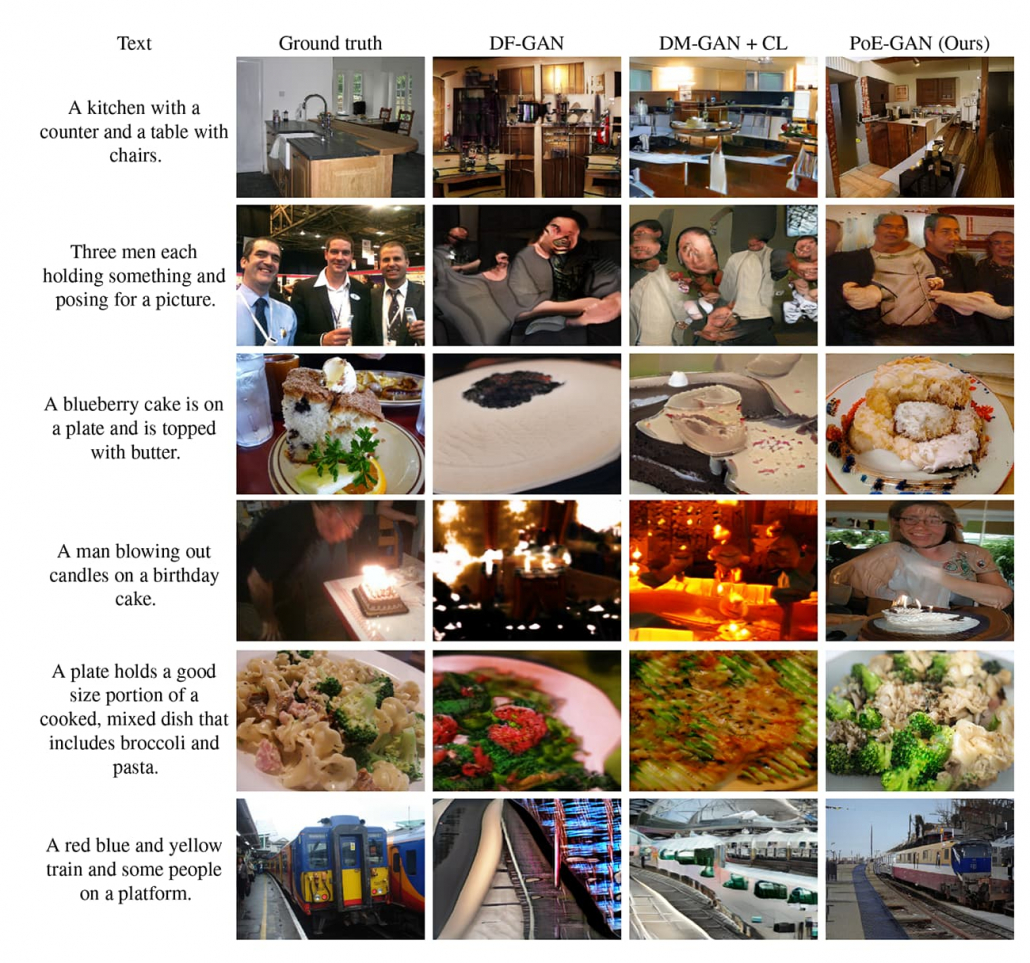
PoE-GAN’s experiments in non-landscape domains improve on SOTA methods such as DF-GAN, but are facing a harder challenge. Source: https://arxiv.org/pdf/2112.05130.pdf
Architecture of PoE-GAN
Relatively little work has been done to date on integrating these three methods of user input into a single image synthesis system, as the paper observes. For the most part, Variational Autoencoders (VAEs) have been used, rather than Generative Adversarial Networks.
As the new paper recounts, research from Denmark in January 2021 also proposed a product-of-experts methodology, and work from 2017-18 from Georgia Tech and Google likewise offered a method of using VAEs to facilitate a multimodal system. Additionally Stanford has a Multivariational Autoencoder offering, originally developed around 2018.
However, the paper acknowledges PoE-GAN’s similarity to M6-UFC: Unifying Multi-Modal Controls for Conditional Image Synthesis, a paper released in May of 2021, a collaboration between DAMO Academy, Alibaba Group, and Tsinghua University. The authors of the NVIDIA paper comment that ‘the way they combine different modalities is similar to our baseline using concatenation and modality dropout’, but contend that their own PoE generator design ‘significantly improves’ upon this work.
The architecture of PoE-GAN requires the orchestration of multiple combative distributions, and the ability to account for and remedy a number of potential failure examples, such as the lack of data in one of the three available modalities, which must fall back to a usable result as the worst case of ‘graceful failure’.
The concept of combining multiple distributions in this way has come to be known as product-of-experts.
You can see further details of the approach the researchers have taken towards the end of the video below, and in the supplementary material for the new paper.
Martin Anderson is a freelance writer on machine learning and AI.